Artificial Intelligence (AI) is talked about everywhere, from its admirable role in cancer diagnosis to scaremongering about robots taking over jobs. Where did AI come from though, and how did it creep up on us all of a sudden?
With a slant towards the UK and Europe, this article attempts to give a holistic view of AI’s journey and enlighten on its breadth and scale. It also intends to dispel some myths, raise awareness about aspects that surround AI, and suggest areas for consideration in the future.
It's been a rocky road
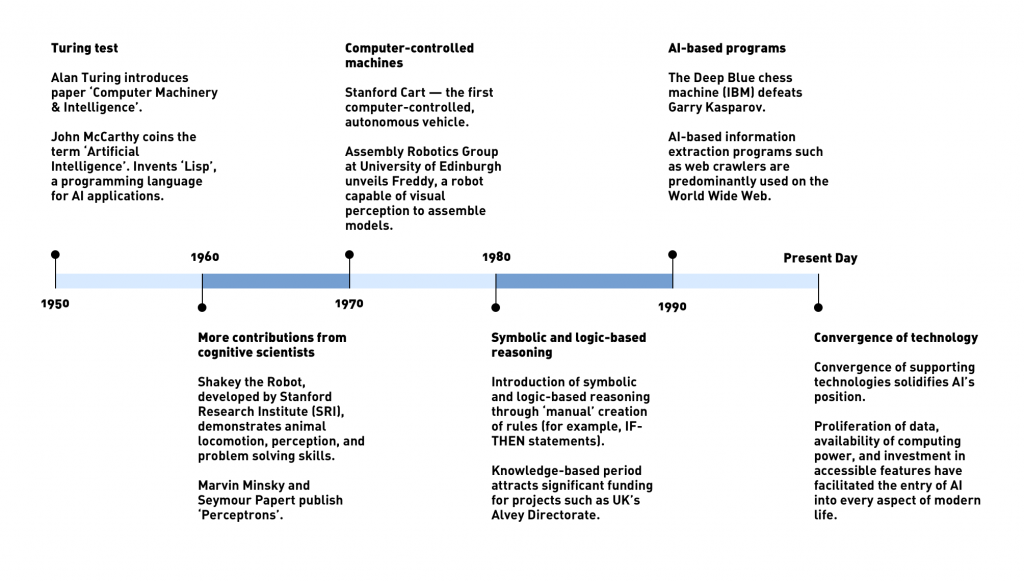
With all the buzz around AI in the last few years, many people would be surprised to know that it has actually been around for over 70 years, starting way back in the 50s with Alan Turing and his now-famous Turing Test (popularized by the 2011 movie ‘The Imitation Game’). This test set the benchmark for intelligent machines for years to come and is still referenced today 76 years on.
The 50s also saw contributions from the likes of John McCarthy and Isaac Asimov who helped bring the term Artificial Intelligence into the public’s imagination through science fiction and research directives.
The 60s then expanded this perception with the popularization of robots (in films such as 2001: A Space Odyssey and for real by Stanford Research Institute, which created SHAKEY, the world’s first mobile intelligent robot) while at the same time dismissed the promise of neural networks1 with Marvin Minsky and Seymour A. Papert’s Perceptrons paper. This dismissal then extended to the rest of AI with the first of what was called an AI winter. Just over a decade after the term AI was coined, rejection set in, with unrealistic expectations and money wasted. AI, it seemed, was dead and left to science fiction writers with little room in the real world.
Was that it for AI? Not so. Expert and knowledge-based systems came to the rescue. The 80s saw the introduction of symbolic and logic-based reasoning through the ‘manual’ creation of rules (for example, IF-THEN statements). Unlike their predecessors, these systems were confined to specific problems, such as diagnosis and classification instead of general problem-solving capabilities. This knowledge-based period in the AI timeline again attracted a lot of funding, including the UK’s Alvey directorate2 and the CYC project.
In time, however, disappointment returned, with the realization of these systems being too static and inflexible when scaled up or adapting to changing environments.
The AI winter was back again with disappointing memories of what is now termed ‘Good Old Fashioned AI’ (GOFAI). What next? Where was AI to go now? Dabbling with ‘brute force’ approaches brought successes with the likes of IBM Deep Blue beating world chess champion Gary Kasparov, but the real promise came in the resurgence of adaptive computation and neural networks.
This resurgence proved fruitful. After many iterations, it brought us to the new millennium, which saw the advent of machine learning, robotics, computer vision, and speech recognition. That is not to say it was an easy ride to the present day, there being many disappointments and realignment along the way. Successes, however, were now real and repeatable, the winning of the jeopardy game show in 2011 being a showcase.
AI was now ready to be used for everyday problems in multiple sectors3.
Look where we are now
While the aforementioned timeline highlights the key events in AI’s journey to the present day, it is worth noting that a major factor in AI’s place today is due to the convergence of supporting technologies. The proliferation of data, the availability of computing power, and investment in accessible features have facilitated the entry of AI in every aspect of modern life.
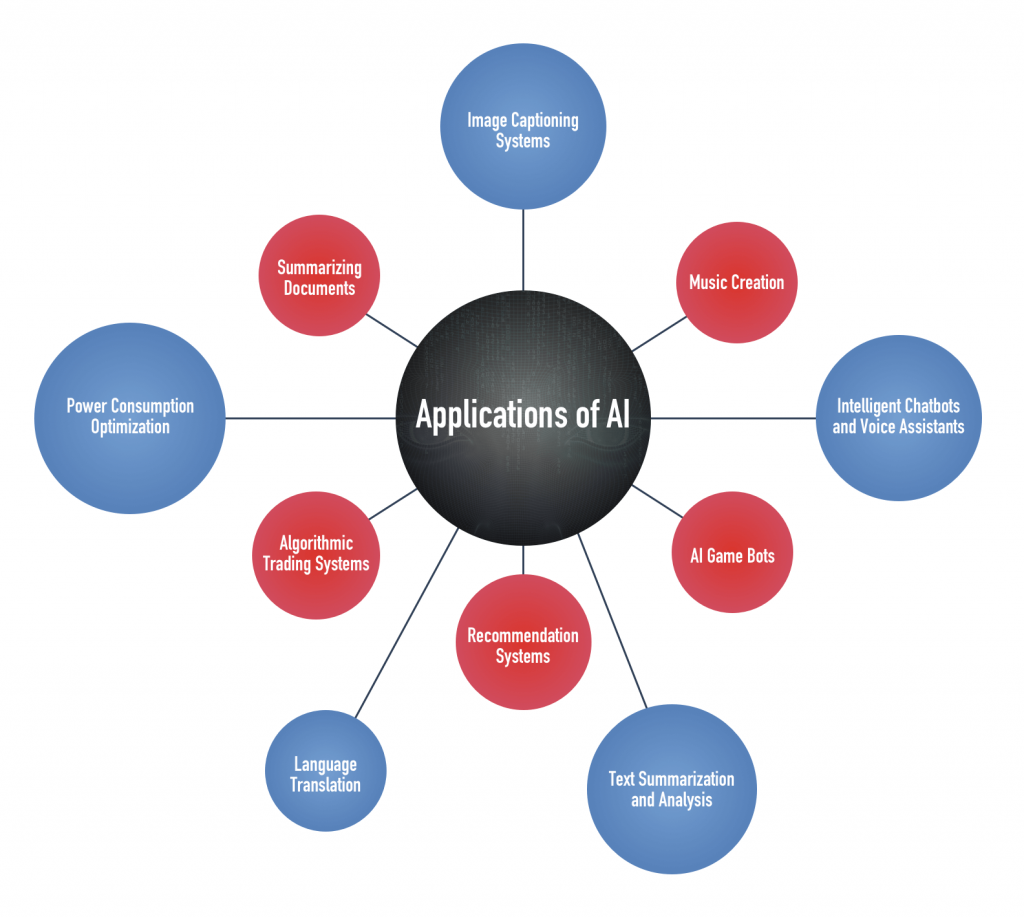
To give an idea of some of the exciting ways in which AI is now part of our society:
- Intelligent Chatbots and Voice Assistants
These chatbots can respond to your queries keeping in mind your personal preferences and the context of the conversation.
- Language Translation
Translation is done by converting sentences into an intermediate representation and then to the required language. This enables more natural translation.
- Recommendation Systems
AI is used to identify content based on your previous preferences. This helps in serving content that you are most likely to be interested in.
- AI Game Bots
Not since Deep Blue beat Garry Kasparov has there been so much excitement about game bots. AI game bots prove that machines are now capable of transcending human expertise.
- Text Summarization and Analysis
Sentiment analysis, text classification, and summarization based on Word2vec model and Long/Short Term Memory (LSTM) networks: Word2vec encodes the concepts represented by words in a high dimensional space. LSTM networks can glean long-term dependencies between various concepts in the text.
- Power Consumption Optimization
The cooling systems of racks in data centers can be optimized based on historical cooling data, leading to more efficient operations.
- Image Captioning Systems
We have image captioning systems and also systems that can generate images based on captions.
- Music Creation
By sampling huge music datasets, AI can create human-like compositions. Also, we have systems now that can recognize music in real time and show lyrics in sync with the music.
- Summarizing Documents
Recent developments in the field ofAI and Natural Language Processing can be leveraged to generate summaries of long articles and even answer questions based on the content.
- Algorithmic Trading Systems
Using AI, these systems can make decisions at the speed of lightning, even surpassing human capability. They do not need human intervention and can analyze real-time data along with historical trends to make decisions.
Rather than adding more and more to this list, which we could easily do, it is useful to instead look at how we classify all these types of AI. There is no better way of showing this than by referencing Francesco Corea’s AI knowledge map:
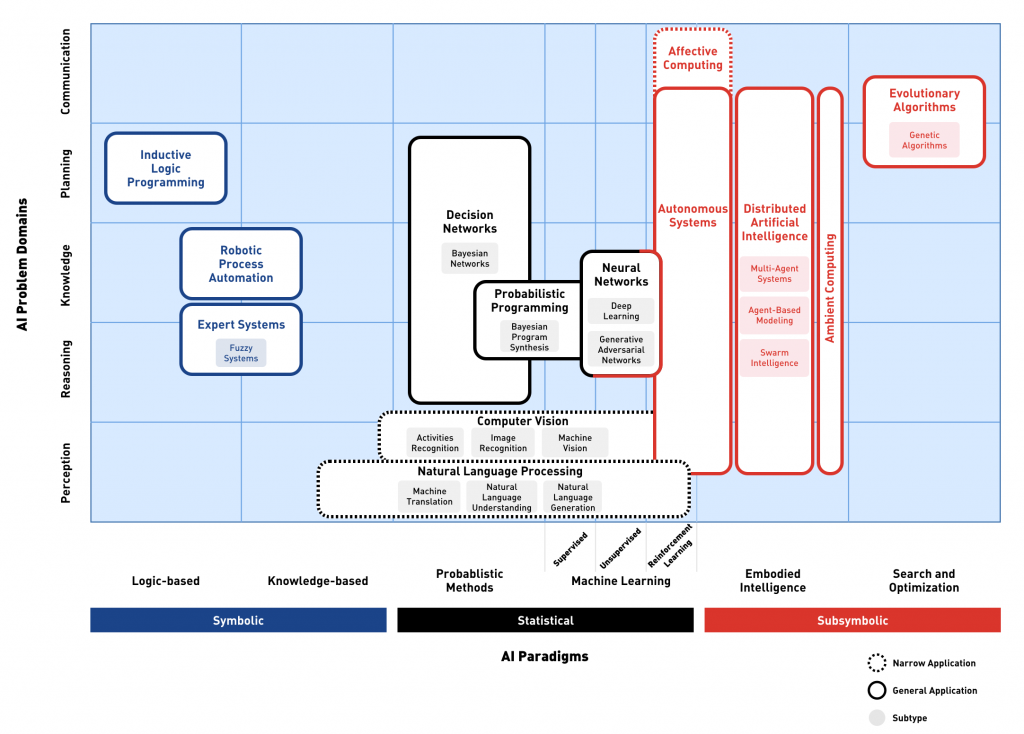
Not only does this create a matrix in which different AI technologies can be placed according to paradigms and problem domains but it also helps to illustrate the wide spectrum of techniques that come under the AI banner, perhaps as enlightenment to those new to AI or those who view it primarily through machine learning glasses4. The source article also highlights the additional grouping of weak and strong AI, which is all about mimicking human behavior, taking us back to some of the original aspirations mentioned in our initial AI timeline above.
“AI's use in a decision support capacity is to aid rather than automate"
Lastly, another grouping which is very important to highlight is that of assisted\augmented versus automated AI. In popular media, AI is projected as automating and taking over, when in reality its use in a decision support capacity is to aid rather than automate. This is an area that will gain more momentum over time, and help avoid repeating previous mistakes.
The future is optimistic and challenging at the same time
AI has a very bright future with promises of:
- Fully Automated Cars
Within a decade, we could have cars that drive better than humans, thus reducing the risk of accidents.
- Real-Time Translation Systems
These will translate without losing the essence of communication, unlike the current crop of real-time translation systems.
- Deep Neural Net Architectures
Architectures such as Generative Adversarial Networks, LSTMs, and Convolutional Neural Networks will work together to provide a form of generalized intelligence. It could be used to generate art that may be aesthetically pleasing to humans.
- Internet of Things (IoT)
By leveraging the huge amount of data captured by IoT devices, neural networks can be trained to mine granular insights and provide accurate forecasts.
- Hardware for Machine Learning
Dedicated hardware that performs faster than traditional hardware for training neural networks will emerge.
- Convergence of Symbolic AI and Neural Nets
A convergence of symbolic AI and neural networks could take place. With an interface between the two, we can get neural nets to memorize certain things and refer to them later. In this way, we could use traditional algorithms in conjunction with neural networks.
- Medical Diagnosis
We could have neural networks trained on medical cases so that accurate diagnosis of medical problems based on patient history will become commonplace.
- Bias Removal
Many machine learning algorithms have become susceptible to bias present within the training data. New techniques can help to deal with these biases in systems.
- Augmentation of Human senses
Advances in neurobiology suggest that the human brain can adapt to various types of novel sensory inputs. We could have machine learning modules that can interface with the human brain to enhance our perception of the world.
- Artificial General Intelligence
A combination of several specialized AI, Artificial General Intelligence is an area tech giants have set their sights on. It could mean systems that are much more autonomous, intelligent, and sophisticated than say, Flippy the burger-flipping robot or Atlas the humanoid.
According to Gartner, 37% of organizations have implemented AI in some form already. This is not restricted to business, with governments, including the UK and Europe, formulating strategic initiatives to leverage AI’s many assets.
While there are lots of opportunities, some have voiced concern over the ability to successfully deliver or integrate. This is down to the fact that AI is currently outstripping the skills currently available to implement. The lack of digital skills, in general, is already well known; the lack of AI skills trumps this considerably. When we consider Forrester’s assessment that by 2020, 47% of revenue will be affected by digital, coupled with the growing concerns around AI automating many jobs and making people redundant, do we need to panic? Not at all!
While AI will replace some jobs, it will actually create more. Considering the potential for AI to enhance existing social and collaborative skill sets, it is somewhat paradoxical to think that technology may actually get people talking with one another more, something that has not been traditionally associated with technology and digital enhancements. We only have to look through history and see how we humans have adapted in previous times of huge change (such as the industrial revolution). If we start to embrace the positives that AI will inevitably bring, it is very encouraging indeed. This is particularly true in the light of an assessment from the McKinsey Global Institute that Europe could “add some €2.7 trillion, or 20 percent, to its combined economy output, resulting in 1.4 percent compound annual growth through 2030” if it pushes ahead on the AI front with its current assets and capability.
“the key will be adaption not replacement”
In order for this transition to be successful, we have to be mindful of a number of key supplementary areas:
- Education
With the potential to have such a wide impact on so many people, it is important that society as a whole is properly educated on what AI really means and, more importantly, how it can help. This will help dispel the fears, concerns, and assumptions that non-technical5 people may have.
- Ethics
We must always consider the ethical aspects along with the technology promises, and it is encouraging to see such developments in this area. Major technology providers have developed guidelines to govern their use of AI. But as technology develops, new risks are bound to appear, raising fresh questions regarding privacy, transparency, and accountability. It is crucial that we remain sensitive to these issues and be open to discussion on questions our customers or other stakeholders may have.
- Accountability
While this is of more importance to certain sectors than others (for example, medical diagnosis in healthcare as opposed to stock predictions in retail), accountability or the ability to explain why and how decisions were made will continue to be of critical importance. We only need to look at the recent Uber incident, which raises questions of criminal liability in the event of an accident by self-driving cars, to remind ourselves of the criticality of this area. It will be interesting to watch the merging of different symbolic and adaptive AI techniques to help solve this.
- Data Privacy
Perhaps an inevitable area for consideration with increasing regulation and privacy initiatives (for example, GDPR) will be that of data privacy.
Conclusion
AI will continue to grow, and it is equally important for the socio-economic aspects to be fully considered as its journey continues (for example, education, ethics, etc.).
It will be interesting to see which sectors and areas will have the most success, and I am excitedly looking forward to writing an addendum in five years time to this article!
Footnote:
- While not actually the first neural network, the perceptron is often cited as the first.
- Thought by many to be in response to Japan’s ambitious Fifth Generation Project.
- For more details see the BBC’s excellent article that shows more details on the above timelines.
- We mean in no way to diminish the power and value of machine learning in recent history, only to highlight the additional members of the ‘AI family’.
- Considering AI’s potential, it needs to be taught with technical features per se, for adoption to be as seamless as possible.
AUTHOR BIO
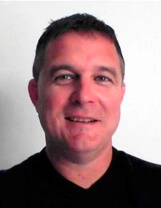
Simon completed an MSc in AI over 24 years ago and since then has seen many interesting changes to the AI landscape. He started as an AI engineer at British Steel Laboratories, working with cognitive templates, and moved onto consulting around knowledge management initiatives. During AI winter\s, he spent his time successfully delivering management and technology initiatives across multiple sectors.
Currently, Simon is Managing Director and Co-Founder of New Leaf Technology Solutions, where he enjoys indulging his passion for AI again. His specific interests include AI in diagnosis, NLP, and the convergence of symbolic and adaptive AI techniques.